Crossing the Chasm to AI Success – Step-by-Step
AI is taking off like a rocket. A recent study finds 85% of companies already have AI projects in evaluation or production. That said, dotData CEO Ryohei Fujimaku cautions any AI investment also need solid forethought. He shares his tips for AI success and ROI.
by Vance McCarthy
Tags: AI, apps, dotData, data, developers, machine learning, survey,
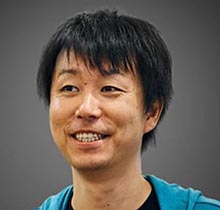
CEO

"Building AI applications because everyone is doing it is a path to failure."
As 2021 kicks off, the rapid growth and adoption of AI and machine learning within the enterprise are set to gain even more steam in the new year.
According to a 2020 report by O’Reilly Group, 85% of surveyed companies say they are already using AI in production projects or evaluating AI. That said, adopting AI isn’t always the same as benefiting from it.
Crossing that chasm -- from evaluating AI to proving it can deliver beneficial ROI – often turns out to be two different challenges, according to dotData CEO Ryohei Fujimaki.
“Building AI applications because everyone is doing it and throwing any problem at AI without concrete objectives is a path to failure,” Fujimaki said. “AI is an incredibly powerful technology, but it is not a panacea for every business problem.”
Indeed, there are signs in 2021 that AI/ML is becoming a new-age hammer, poised to pound at everything that remotely looks like a nail.
To help companies be more discerning with their AI budgets and staff, IDN asked Fujimaki what AI tasks are best.
“AI is great at sifting through massive amounts of data, discovering patterns, and finding hidden insights that otherwise are not obvious,” Fujimaki told us.
Fujimaki also offered advice to business stakeholders considering an AI investment.
“Start with clear, quantifiable business goals,” he told IDN. “Businesses must have straightforward ways of measuring not only if the AI solution is doing its job, but whether it’s doing a better job than was being performed without AI – and by how much. It’s only with such clear, quantifiable goals that enterprises can fully leverage – and benefit – from investments in AI.”
Sound advice, but frankly this can be easier said than done. Often, AI projects can turn out like this.
When developers run these projects, a business ROI is frequently murky. On the other hand, when business execs try to set such goals, they misapprehend AI capabilities or timelines involved with such projects.
dotData’s Fujimaki Shares His Step-by-Step for AI Success
So, we asked Fujimaki to share his expertise and recommend some step-by-step advice for those embarking on an AI initiative.
The first step. To get started, AI leaders should prioritize hard to solve complex business problems that have clear objectives. They should assemble a cross-functional team of technical and functional experts, ensuring buy-in from domain experts.
As an AI project champion, you can set plans to succeed by asking the right set of questions:
- What pain points will this initiative address? Define the business problem and key business interests.
- Can this business problem be mapped to a data science problem? Define clearly data science objectives and identify ML problem category
- What are the business and data constraints? Formulate data analytics strategy accordingly.
The next step is to define success criteria and translate data science metrics to business metrics. If companies can monitor the business metrics before the AI project started and after implementation, they can easily quantify the impact, he said.
Fujimaki shared with IDN a detailed example:
Let’s assume that your business problem is related to churn analytics, a common use case for online subscription-based businesses or any online service vendor.
You need to collect data about users that have canceled and renewed their subscriptions during the past three years. You are interested in lowering the customer churn rate by analyzing your customer behavior to determine the common characteristic of churning customers. The data science problem then is to predict whether or not customers will cancel their subscriptions. The key points to think about are:
How to decrease the customer churn rate to meet the business objective?
How to identify customers with high chances of churn to target a promotional campaign?
How to monitor the efficacy of this campaign strategy in real-time from a business revenue perspective?
Build a binary classification model to identify if a customer is going to churn away.
○ Yes - high risk, need further action.
○ No - low risk, no action for now.
If "churn rate" is the metric that the business uses to track performance, then the metric should be tracked before AI project implementation and after implementation. They are designed to provide precise and easily interpretable models of the rules used in any given ML algorithm.
Fujimaki said these steps taken together will provide all AI stakeholders -- model developers, model consumers, and business teams – the full confidence and certainty they need to execute complex data science projects.
“Clarity offers business users a more straightforward path to understanding how an AI algorithm works and the relationship between data elements that allowed the algorithm to arrive at a specific use-case,” Fujimaki told IDN.
Related:
- Agentic AI: Unlocking Business Value with the Next Evolution in Enterprise Intelligence
- Zilliz Improves AI-Optimized Vector Databases with More Speed, Scale and Ease of Use
- Informatica, Snowflake Team Up To Speed AI App Delivery; Simplify Data Management and Governance
- Gartner: AI-Augmented Development Hits Radar for 50%-Plus of Enterprises
- OutSystems AI Agent Builder Brings No Code Development to GenAI
All rights reserved © 2024 Enterprise Integration News, Inc.