Understanding Your Data Sprawl Across Clouds and Hybrid Enterprises
As enterprises recognize the value of their data ecosystem, thy are taking a next step. Arthur Shectman, CEO of Elephant Ventures explains why modern lean product development practices can result in better understanding of data – and drive valuable data-driven decision making.
by Art Shectman, Founder and President of Elephant Ventures
Tags: cloud, data, hybrid, IoT, lean, streaming,
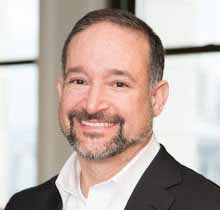
President
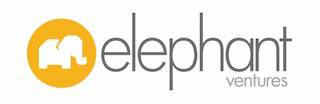
"When you take an objective look at the tidal wave of data organizations have access to, the volume of unused data is truly staggering."
Seagate’s 2020 report, “Rethink Data” (a global survey of tech decision-makers in business) states ‘no company wants their data lake to turn into a data swamp where unleveraged yet potentially useful data sits dormant’.
The new reality for IT teams is that while most businesses have invested heavily in hybrid and multi-cloud strategies, to house and manage their data infrastructure and data acquisition, the business also frequently struggles to understand the nature and potential use of that data.
With volumes of data sprawling across multiple clouds, IoT networks, on-premises hardware, and external social and mobile applications, when you take an objective look at the tidal wave of data that is arriving moment to moment, or the new data that organizations now have access to, the volume of unused data is truly staggering. As a result, much of the latent value in the data does indeed lie dormant and so most businesses struggle greatly to capture value from it.
When businesses try to evaluate the ROI for their hybrid cloud investments, the supporting costs of data acquisition and storage, and the expense and human resources needed to power their analytics and data science programs, they often find lackluster results or outcomes that are built on false recommendations.
In turn, they frequently turn attention to IT processes or spend and try to isolate what to cut, and where their process quality issues might be a blocker to capturing business value from their investments. They often reorganize or introduce new management in their IT teams, and yet still struggle to unlock the full potential from their data and data infrastructure.
Unlocking Data Value Using Modern ‘Lean’ Practices
In these environments, companies experienced in “lean product development” practices may have the ability to better understand the expanse of their data under the lens of their overall corporate priorities.
This is because such firms tend to invest in leveraging design thinking practices that ask “what might be possible?”, and spend short, focused bursts defining a clear scope, researching and documenting precisely what data can be available to them. They also tend to involve the end-user analytics or business teams in what are traditionally IT buildout or tech operational programs.
These companies are also well-positioned to rapidly capture value from data-driven decision making by assembling and exploiting the full breadth of their available data catalog.
Organizations should first work to build their hybrid cloud strategies on a robust data foundation from the ground up. Enterprises must have a holistic approach where they merge an integrated data architecture that aligns data storage and access with an active and up to date data catalog that is framed or organized along the dimensions of their core domain models of business objects, matched to their business needs and tagged with relative risks assessments of the data from the perspective of its source and its intended usage.
Once the basic systems and data catalog are in place it is helpful if the IT teams take a progressive build out technology to support a progression of data usage from the business.
Stages for Helping Your Enterprise Deliver Valuable, Data-Driven Decisions
The progression of data internalization and use at a high level matures in these general stages:
Exploratory - gaining an understanding of what’s there, gaining access to it, documenting, and understanding the relationships in the data to your other organizational data stores through internal or external keys.
Directional Decisions - making broad directional business decisions from data, custodial data processing becomes necessary. Data must be clean for basic queries. Real entity resolution becomes necessary.
Financial Decisions - models predict specific actions and outcomes that directly impact the financial health of the business, quality at the data-set level and field level become important. Compliance or root cause analysis typically require data provenance systems to be in place.
In the exploratory stage, establishing access is key. Leveraging strong organizational identity and entitlement tools can be very helpful to accelerate this process. Teams should take care to be sure they are authoring shared hygiene libraries and to publish a shared mapping of their data into a centralized and accessible catalog to help speed explorations and to expand the overall understanding of what might be in the data. The early indicators of success are the number of shared tools and the reuse of the outputs of your data teams see.
In the directional decision-making stage, it is critical to prioritize your actions in the strategic prioritization framework of your overarching business priorities and to use that framework to decide what is the next most important problem to tackle. The result should be insight into which part of your data ecosystem to get ready for analysis, or which hole in your available data to fill in next.
Notably, in this stage of maturity, the processing load of active production data hygiene and curation tasks is to need more processing capacity and supporting elastic infrastructure. Orchestrated, elastic data ops and DevOps become important to reduce cycle times and to keep costs contained for large data sets, and at times infrequent or burstable calculations.
Finally, as core financial decisions begin to be integrated into production pipelines, active alerting and monitoring become important to increase the reliability of your decision making and analytical forecasting services. Periodic reprofiling of your data becomes important to capture drift in systems and data that might invalidate historical system outputs and model outcomes.
While these supporting steps are common, perhaps the most important concept for ensuring that business value is captured is to regularly align technology and business stakeholders along this journey.
Take a page from the lean product development book and get end-users and experts in the room early, ask about the business’ ability to act on the outcome of any analysis and the impact of the analysis is wrong to surface risks.
In addition, use those conversations to prioritize the work of your internal IT and data support teams alongside your data science team’s priorities and overarching business priorities. By taking a realistic look at what is possible, and what is strategically important, you can look for wins across initiatives at first, and accelerate your time to capture the value and optimize the costs associated with each incremental subsequent initiative.
Art Shectman is Founder and President of Elephant Ventures, a digital innovation and agile/lean product development and engineering firm based in New York City. He is a digital architect and strategist with more than 25 years of experience spanning a wide range of sectors, including intelligent robots, automation, adtech and secure trading networks.
Related:
- Agentic AI: Unlocking Business Value with the Next Evolution in Enterprise Intelligence
- Zilliz Improves AI-Optimized Vector Databases with More Speed, Scale and Ease of Use
- Informatica, Snowflake Team Up To Speed AI App Delivery; Simplify Data Management and Governance
- Gartner: AI-Augmented Development Hits Radar for 50%-Plus of Enterprises
- OutSystems AI Agent Builder Brings No Code Development to GenAI
All rights reserved © 2024 Enterprise Integration News, Inc.