MapR Adds AI/ML To Its Converged Data Platform To Deliver Faster Insights, More Automation
MapR Technologies is enriching its MapR Data Platform with a set of AI and machine learning services to speed analytics with a broad range of automation and efficiency features. MapR’s latest update sports AI-powered goodies for most analytics stakeholders -- developers, admins, IT operations, business users and even data scientists.
by Vance McCarthy
Tags: AI, analytics, autonomous, data, machine learning, MapR, pipeline,
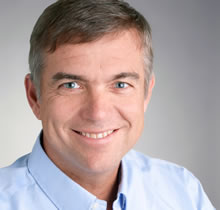
SVP Data and Applications
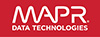
"We see the advances of AI/ML, combined with our unified data platform, will speed the operational impact of analytics."
MapR Technologies is enriching its MapR Data Platform with a set of AI and machine learning services to speed analytics with a broad range of automation and efficiency features.
“In this update, there are 30-35 features that are pushing the platform forward. AI and machine learning represent one of the biggest steps in this evolution,” Jack Norris, MapR’s senior vice president for data and applications told IDN. The latest updates to MapR’s converged data platform represent the widest and richest set of AI and autonomous capabilities yet to be added, he said.
Taken together, a review of MapR’s latest update reveals AI-powered goodies for just about all major enterprise stakeholders -- data scientists, developers, admins, IT operations and even business users.
“This latest update has AI features that can apply to each stage and many different people,” Norris told IDN. “We see the advances of AI/ML, combined with our unified data platform, will speed the operational impact of analytics through automation and self-adjusting capabilities.”
MapR’s take on AI/ML brings a range of benefits - from self-managing, self-service and even self-healing, he added. This is mainly because MapR is taking an ‘end -to-end’ approach to AI/ML.
As Norris put it, rather than simply focusing AI on end-point analytics, MapR is bringing AI/ML to the full pipeline. The results: smarter storage, more responsive management, automated tasks and fast analytics app development.
It’s Time for AI/ML, As Today’s Data / Analytics Infrastructure Comes Under Strain
MapR’s investment in AI/ML comes as they are seeing customers suffer as their traditional data pipelines crack under dual strains, Norris said. These strains are: mounting data volumes and the need for more speedy results.
“There's a growing list of jobs that just can't be handled with existing clusters,” Norris told IDN. “Not only do we have Hadoop and Spark jobs going through on the HDFS API, you’ve got the new generation that are going through file access directly. On top of that, you've got containerized projects that are being shared across the organization. With a traditional approach, not all of those can be serviced well by the same underlying platform.”
Fellow MapR exec Anoop Dawar, MapR’s senior vice president product management and marketing, laid it out this way in a statement. “Customers have made it clear that traditional approaches to managing and processing data for AI and Analytics leave critical gaps,” he added.
AI/ML enhancements to its “comprehensive data fabric” are meant to respond to these gaps. Even before the AI/ML enhancements, MapR allowed NFS, HDFS and S3 to access the same underlying data set. This eliminated the need for duplication and maintenance of separate silos of data. Now, after several years of experience, MapR engineers believe it’s the right time to bring AI, ML and autonomous services into the mix.
“There’s no free lunch in terms of processing the data. So the simpler you can have the data available, the simpler that the platform can manage that data,” Norris said. “At MapR, we've collapsed the time delay, so now users can have low-latency, complex operations that are manipulating the data.”
The AI/ML enhancements will make MapR’s entire data pipeline and analytics environment even smarter, faster and self-maintaining, Norris told IDN. “Our proven platform capability, we feel is what's really required for AI/ML to show some very interesting results – in a time-frame that’s very compressed,” he added.
MapR’s Approach To AI/ML Aims To Help a Wide Range of Analytic Stakeholders
MapR’s addition of AI/ML capabilities could hold promise for a wide number of stakeholders across any analytics projects – developers, IT operations, security admins, data scientists and business users. Here’s a quick summary:
For developers, MapR’s AI/ML capabilities aim to make it faster and easier to design, build and deliver analytics apps. One of the biggest barriers to developers working on analytics apps, Norris said, is all the back-and-forth that often happens to get access to data and models. Developers often find they need to go all around to ask for permissions from many people – data owners, security and even data scientists themselves.
“As a platform provider, we want to make the data readily accessible, manageable and usable within the system,” Norris said. So MapR is using AI/ML to automate best practices and important policies, such as security and permissions, he added. AI/ML makes it simpler, even automated, for developers to get governed and permissioned access to the data and models they need. Another boon to developers is MapR's Data Science Refinery, a “containerized, visual space” to make it easier for data scientists share models with both developers and operations.
For IT ops and admin managers, AI/ML-powered features provide smarter and more efficient management of cloud storage. This is mainly thanks to an AI/ML-driven feature MapR calls “object tiering,” Norris said. With object tiering, AI helps create and manage automated, policy-driven ways to place data in the most appropriate (and efficient) location. In practice, this works by automatically calculating the best tier for storing data based several factors (how quickly users need the data, business value of data, performance requirements, etc.)
“With AI, we can optimize for performance,” Norris said. “The system decides to store and write the data is independent of what a developer and administrator does. So we're going to capacity optimize the data. All of that is handled by the system based on the usage information we have and the machine learning intelligence to figure out.”
Business users also benefit from AI/ML in a number of ways. AI/ML combined with MapR’s primary data platform architecture to gain rapid access to multiple types of data -- historical, real-time and streaming. All these data elements can be quickly turned into insights or events. Also, users can glean outcomes from data across on-premises data centers, clouds and edge deployments. The results can be prepped for humans (dashboards) or IoT follows (events and triggers).
Security admins can also get a boost from AI/ML. MapR’s platform offers volume-based data encryption-at-rest, which uses AI/ML to prevent unauthorized access to sensitive data. Further, autonomous secure file-based services ensure on-going compliance with NFSv4. Added encryption avoids exposure to threats such as packet sniffing or even and theft of storage devices.
Even data scientists, who often love their access to “raw data” get some AI/ML benefits. They can leverage AI/ML to test, share and distribute their models and other work to others.
MapR Says AI/ML Supports the Paradigm Shift for How Customers Want To Use Analytics
Norris notes AI/ML capabilities go beyond smarter systems and automated tasks. AI/ML, along with MapR’s converged data platform, will bring together two traditionally competing analytics architectures – and offer a third way.
“By adding AI and machine learning to MapR’s architecture, the company’s platform is able to bring together two of today’s most popular approaches,” Norris said. Those two approaches have often been an ‘either-or” proposition. They are:
(1) a focus on the underlying platform architecture and
(2) a higher-level focus on data-centric pipeline models that actually deliver business insights from raw data.
As neither approach on its own can do everything customers need, “we're seeing customers going through a paradigm shift,” Norris said. So, to help we’re bringing both models together,” he told IDN.
“The old model was ‘You needed a pipeline, so you need an ETL transport, then transform the data, put it in the right kind of construct.” While that’s been a very popular model, the downside to this, Norris said, is that only after all that prep work could companies do analysis on the data or use it in other ways to support their application processing.
This model is seeing some cracks, creating cracks and fissures. “This model worked for a long time,” Norris said, “but today we’re seeing that model is breaking under the weight of exploding data volumes and the need for faster results and less delays.” In fact, a report this spring from Gartner also found traditional data pipeline approaches are coming under increasing strain. In part, Gartner’s 2018 Strategic Roadmap for Compute Infrastructure," (Apr 2018) found:
“Data growth has far outstripped compute growth, resulting in an imbalance in system architectures. Emerging data-intensive workloads that require data-centric processing — such as AI, high-performance computing (HPC) and IoT — will expose the system imbalance, especially in data movement, resulting in new architectural innovations to address this gap.”
So, to address these cracks, MapR’s model for doing analytics will “provide infrastructure and services that provide a more rapid access to insights,” Norris said. The shift in thinking really comes into focus in this respect, Norris said.
“The main idea is to stop thinking about architecting discreet data silos.” Instead, the pivot in thinking is to focus on how to drive analytics and insights by providing simultaneous access to all three major types of data – (a) historic activity, (b) transactional and customer activity data, and (c) just-in-time acquired streams. “While many [users] agree for the need for such a model, it takes some thought and design work. It also can cause customers to rethink how they can use all their data,” Norris noted.
The key to truly getting max value from AI/ML in analytics is to marry those technologies with a platform that can leverage both the traditional and new approaches. AI/ML plus a flexible, full-featured platform, Norris suggests, allows for a host of more “dynamic approaches” to analytics.
This focus on dynamic analytics also leads one to craft a new equation for AI/ML:
Flexibility plus dynamism is a basis where AI/ML services can truly power new-gen apps, storage, triggers and even security – and set the stage for innovation and business differentiation.
Integration Developer News will continue to monitor how AI/ML is powering the next-waves of innovation and disruption.
Related:
- Informatica, Snowflake Team Up To Speed AI App Delivery; Simplify Data Management and Governance
- Gartner: AI-Augmented Development Hits Radar for 50%-Plus of Enterprises
- OutSystems AI Agent Builder Brings No Code Development to GenAI
- Next Pathway Leverages AI, LLMs To Speed, Simplify Cloud Migration Projects
- Gartner Says Only 12% of Infrastructure & Operations Leaders Exceed CIO Expectations
All rights reserved © 2024 Enterprise Integration News, Inc.